Startup Spotlight: January AI
Startup Spotlight is an interview series where we ask health, fitness and wellness startups that use Terra, to share their wisdom from their own journey to success and also where they see fitness data going in the future.
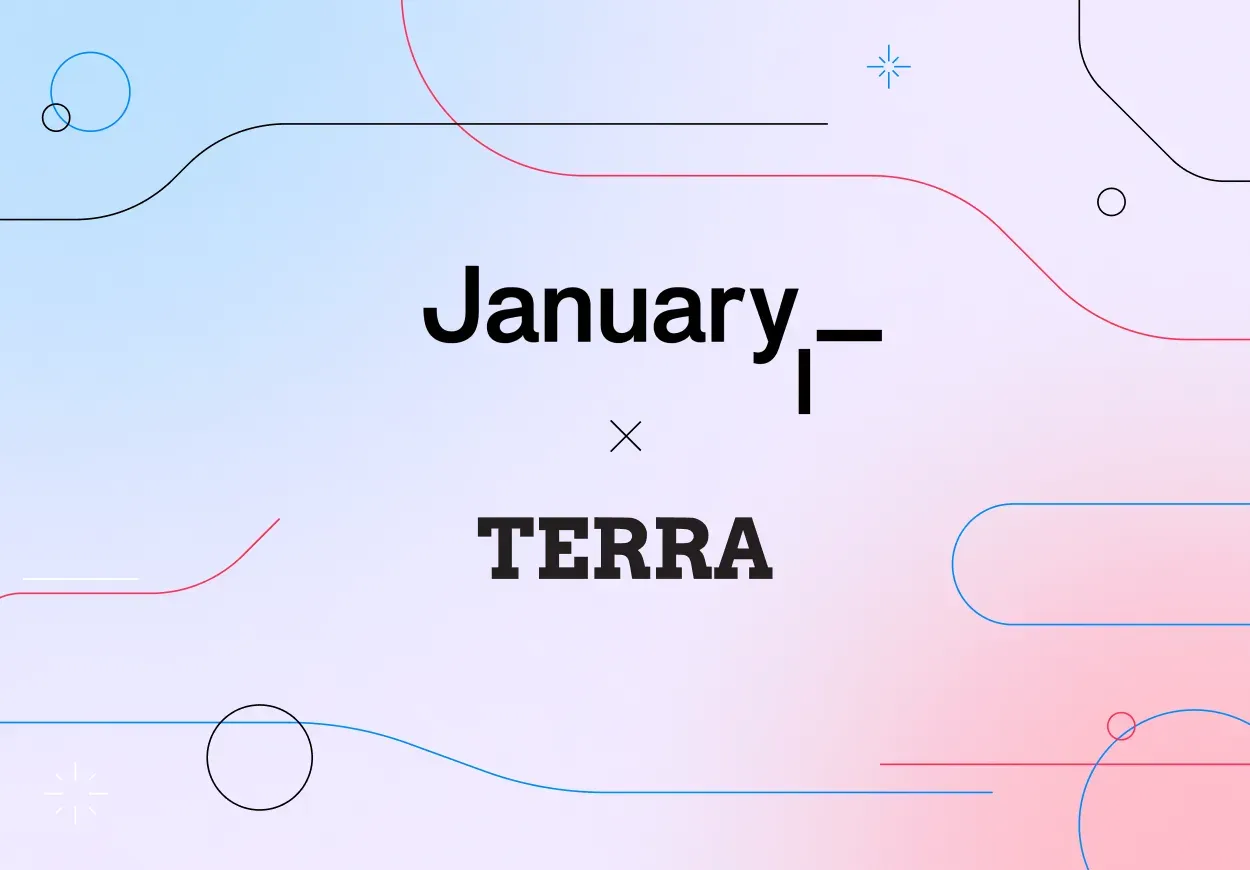
In this Spotlight, we connected with Maher Hanafi, Vice President of Engineering at January AI, the platform that uses AI to predict how an individual's glucose reaction will be and after a period of training these predictions are accurate without a CGM. Their goal is to help people evaluate their health ahead of complications and ultimately, flip the whole healthcare system upside down. Check out more below!
Describe your company:
Maher Hanafi (MH):
January AI is a platform that uses different sources of data including CGMs such as the Freestyle Libre and wearables such as the Apple Watch, Fitbit, and the Oura. CGMs give us blood glucose and all the other wearables give all sorts of biometrics.
We then use this information to inform people how their bodies are reacting to different things. Our main focus today is on food, and we want to tell people how their glucose levels are changing based on the food they are eating. On our product we have a food logging experience with a database of more than 20 million items. When people log their food they can customise their food and what they eat - understanding this data and collecting their glucose real time we can understand how their bodies react.
The uniqueness or value proposition that we have is in the name - it's our AI. We use AI to predict how the glucose reaction will be after a period of training. People will use the sensors and devices for a couple of days of training, and after that even if you don't have a CGM on you we can tell you how your glucose will react based on the food that you are eating. This is the result of two years of research, trials, and white papers.
For example if somebody is interested in eating something we can look at their data and say "That might be healthy for others, but not for you based on the training and based on how your body reacts to food like this - it could cause your glucose to spike really high." The beauty of this also is that we have a huge database of all the restaurants available in the US. You can use our app to look at menus and figure out what you want to order - we can suggest and recommend food items from the menu for you, we will recommend what is healthier.
We are also working on building better recommendations and suggestions for people over time, not just based on the healthiness of the food. For example not all healthy foods are things that you would be interested in. Our AI and training is not stopping, it is improving over time to make better suggestions for people.
How did you come up with the idea?
MH:
The idea came from Noosheen Hashemi, a thought leader in the space of metabolic health and lifestyle issues, - in the last couple of years she was thinking about what kind of product she can help build and bring to customers relating to a very important aspect of their life. Health was the main focus, and that was how she met Mike Snyder, the co-founder with a strong scientific background. They both agreed that there is a path here, looking at lifestyle issues - the way we eat, the way we sleep, the way we work, stress, etc. These are things that are preventable. They came up with the idea of preventable health issues and they wanted to put together a system or product in place. Using CGMs was the most important link, but the tip of the iceberg really. They wanted to focus on that first, and then eventually bring in AI to make it truly scalable and really flip the health system from being reactive to health issues to being proactive and preventative. I think AI has to be a part of this sort of transformation. This also comes from the understanding of the costs of healthcare and CGMs - popular CGMs are valid for just two weeks and cost a lot of money, so to have this on someone for an entire year would be very expensive. Using AI to consume and process data from all different sources and being able to log food is the way to go. At the end of the day we need to know more about users so that we can help them with their health issues and also their health journeys, and flip the whole system around to be more preventative. In a multiomic approach collecting data from different sources can help us understand our users better.
Most of our customers are not necessarily diabetics and most are actually optimisers - people who are looking to optimise their nutrition, sleep, and overall well being. Our idea is to build something on top of all the data that is collected. Wearables are more and more popular and the data that is flowing through these systems is huge. All of the data should serve something beyond just graphs, and showing you how you slept. It should be used to make predictions about how you're going to do and feel, and enable us to recommend and suggest alternatives.
What have been some of the biggest challenges you've faced so far?
MH:
One of the biggest challenges that we faced was to be able to connect to wearable devices. When I joined 5 months ago, we only had Apple Watch and Fitbit integrations, that was it. That's why one of the most important challenges to solve for us was to find something that would give us data from the providers very quickly and take ownership of the pipeline - this is where Terra came in. This was a no-brainer decision.
Another challenge is the AI aspect. AI is hard, machine learning is hard. To really be able to train these models to predict is tough. After the AI training is done with specific food types like broccoli, pizza, coffee if one day you wanted to eat something like a caesar salad something completely different, then the AI would be able to predict with a lot of accuracy how your glucose level would react. This was really interesting but also a very challenging thing to be able to achieve, and it took a long time.
Once we had the AI model and machine learning in place, we then had to figure out how to make it into a product and determine which data would be most accurate to refining the training. This is an ongoing challenge. There is no team out there that is perfect at refining machine learning algorithms. I would say productizing machine learning into a software product has been a real challenge for me and from an engineering leadership standpoint we have to put good systems in place. This is because in machine learning you cannot estimate based on the outcome, you can only timebox the training to e.g. two weeks and see what sort of findings are generated from that.
What outcomes have your customers experienced?
MH:
Nutrition has been our number one customer focus - we call them optimisers, people who are really looking at what they're eating and optimising for it. You and I can eat the same chocolate bar and one of us may have a spike in glucose and one of us may not. Increasing the knowledge for these customers about their own health and helping them to prevent health issues from rising from the beginning is one of the biggest pieces of feedback we've received so far. Overall people are very attracted to and engaged in the product and want to change their behaviour and change the food that they eat. They like being able to see how their glucose responds in the app to certain foods, it's like a game.
What do you think are the current gaps in health data today?
MH:
The gap was, and still is, to be able to get all the data to be collected, treated, analysed and to generate a report at the end of the day. If we can track how somebody works out, how they sleep, how they eat, and how their overall health and biometrics are including their heart rate and oxygen levels, and glucose obviously, we can build a dashboard for this. But just visualising this is not enough, we need to build software on top of this and say "based on your genetics, and background, and other factors then you fit ‘here' and we think the best things for you are to follow ‘that' nutrition plan". Everything that is a factor of our lifestyle is what we are trying to influence. The gap is to have as much data as possible.
I know that on our science team we are looking for ways and devices to collect new types of data. Everybody is out there trying to find the next data that can be collected, ketosis is one of these for example. I think athletes have a lot of other things they are interested in looking at, they want to understand a lot of biomarkers to extreme levels of detail. I think insulin would be very interesting to measure. If we have these data points available we can really flip healthcare upside down and bring this to the masses, to the consumers and really help people to see and evaluate their health ahead of time, before it's too late.
Apps too are important, like MyFitnessPal. That's another area - we don't want to be the next app that you need to log your food in. We want to be able to connect to other apps and have it in sync - think of it like Apple Health Kit but for food logging, centralised and shared across services. I think that's it, I think food logging is one of the most important features but one of the most challenging ones because of the complexity. For food there is no system in place that can tell you what your food is, if you don't log it. It needs to be simplified, mainstream and centralised.
Anything else we should write about?
MH:
Would love to give feedback about Terra - I've used other systems in the past that are more established and bigger but for Terra it was very clear and straightforward, the webhooks are great. Your approach to PR and branding is very strong, the next level for you is to focus in terms of compliance - that's how you will grow quickly in the US.